AI Hallucinations: The Limitations of Large Language Models (LLMs)
- Weave.AI Team
- Apr 24
- 5 min read
Updated: 5 days ago
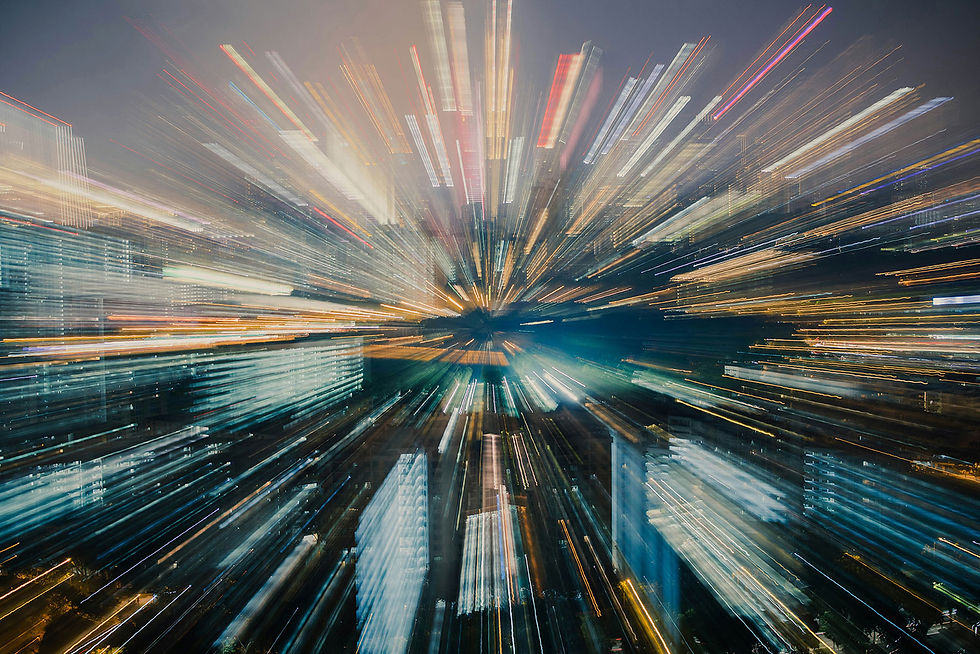
As millions of people adopt ChatGPT and other large language model (LLM)-based technologies to get information, many are noticing that the responses they get back are not always accurate. In some cases, these responses can be dangerously misleading or entirely false.
This phenomenon, widely known as ‘AI hallucinations’, occurs when a LLM generates content that is factually incorrect or detached from reality. Despite advancements in AI, hallucinations remain a persistent challenge—even among leading models like Bard and ChatGPT, which have been found to hallucinate as frequently as 25% of the time or more.
The risks associated with false query results are particularly pronounced for analysts and investors at financial institutions who rely on LLMs to inform critical decisions around investment strategy and portfolio optimization. Hallucinated outputs can result in flawed analyses, misguided investment strategies, and regulatory non-compliance–potentially leading to significant financial losses and legal exposure. As a result, trusting the accuracy of AI-generated insights becomes a major challenge in contexts where precision, reliability, and compliance are critical.
What is an AI Hallucination?
AI hallucinations are responses to a query on a LLM that are factually incorrect, misleading, or not grounded in reality.
Unlike a traditional search engine, which produces links to content, an LLM search produces a summary that pulls information from various data sources. The LLM summary attempts to deliver an answer, even if it lacks correct or complete data. An LLM doesn’t always evaluate the data correctly, yet it still delivers the answer with confidence.
Instances of AI hallucinations can take many forms, including:
Incorrectly asserting that a company operates in a sector or region where it has no presence.
Recommending investment decisions that may be illegal or violate regulatory guidelines.
Misrepresenting a company’s product timeline by suggesting that an unreleased product is already commercially successful.
Identifying a high-risk opportunity as low-risk, leading to potentially harmful business decisions.
Some hallucinations are obvious–such as a result that claims a company has had an IPO when they clearly have not–while others are much harder to spot, such as misinterpreted financials or the results of a growth projection.
How Hallucinations Happen
AI hallucinations arise from a combination of factors inherent to how LLMs are trained, process information, and generate responses. The way LLMs analyze data, which often lacks context and requires the model to make logical leaps, can lead to inaccurate, misleading, or entirely fabricated responses. Again, these responses are often delivered with an unwarranted level of confidence.
Key Factors Contributing to AI Hallucinations
Inaccurate or incomplete Data - LLMs are completely reliant on the information that they are trained on. If they have access to data that isn’t correct, or doesn’t provide the entire story, their results will not be correct either. The popular data idiom ‘garbage in, garbage out’ is particularly true when it comes to LLM query results because they synthesize an answer rather than providing links or sources for the user to analyze themselves. Because of this, the AI model will take the data they have as the complete universe of information and compose a response.
Lack of Nuanced Analysis - A LLM is not designed to gauge the certainty of its response as effectively as a human. If it finds a source that says something, it believes it more or less at face value. That source could be exaggerated content like greenwashing, or an overly negative article written by a competitor. The content could also be outdated, or worse, yet, the LLM believes it because it’s part of the LLM’s training data set.
Faulty Reasoning - A human is able to detect whether there are missing pieces of information and leave those gaps intact. For example, rather than suggest something risky, an analyst will say that they are unable to judge (such as the revenue potential of expanding into APAC) if there is not enough data to make a smart decision. A LLM attempts to fill those holes, and that becomes problematic. A LLM will make an attempt to answer a query and conclude something completely false or impossible with no confidence interval attached.
Ultimately, these limitations make LLMs prone to hallucinations—responses that may appear authoritative but can introduce significant risk when relied upon in high-stakes decision-making scenarios.
Strategies for Avoiding Hallucinations
To prevent hallucinations from compromising high-stakes decision-making, enterprise leaders must focus on three critical elements:
Contextual Verification -AI outputs should be cross-validated against structured, verifiable data sources to ensure that generated insights align with ground truth. This prevents the propagation of errors caused by relying solely on pattern-matching algorithms that lack contextual awareness.
Logic-Based Reasoning -Systems must incorporate symbolic reasoning to apply domain-specific logic and constraints that prevent AI from generating conclusions that violate known business rules or relationships. By integrating symbolic reasoning, models can flag inconsistencies that neural networks might overlook.
Auditability and Traceability -Ensuring that every AI-generated decision can be traced back to its source data provides a critical safeguard against hallucinations. Transparent reasoning pathways allow analysts to assess the validity of AI-driven insights, reducing the likelihood of unverified assumptions influencing business decisions.
Mitigating Hallucinations with Weave.AI’s Neuro-Symbolic GenAI
As organizations increasingly integrate Generative AI (GenAI) into their decision-making workflows, mitigating the risks associated with AI hallucinations has become a top priority. Hallucinations—where AI systems confidently produce plausible but false or misleading outputs—pose significant risks in enterprise contexts, including financial losses, regulatory penalties, and reputational damage. Identifying and preventing these hallucinations requires a more advanced AI approach that combines the strengths of neural networks, symbolic reasoning, and knowledge graphs to ensure outputs are not only insightful but also verifiable and traceable.
Weave.AI: Addressing Hallucinations with Neuro-Symbolic GenAI
Weave.AI’s Neuro-Symbolic GenAI architecture was purpose-built to address the inherent limitations of traditional LLMs by seamlessly integrating neural networks, symbolic reasoning, and structured knowledge graphs. This multi-layered approach mitigates hallucinations by grounding generated insights in verified data, ensuring that every conclusion is not only explainable but also validated against domain-specific knowledge.
Knowledge Graph-Driven Contextual Reasoning
Weave.AI leverages structured knowledge graphs to contextualize and verify AI-generated insights. These knowledge graphs establish explicit relationships between entities such as companies, industries, regulations, and technologies—ensuring that any assertions generated by the AI system are cross-checked against established facts. For example, if a neural model claims that a company operates in a highly regulated jurisdiction, the symbolic reasoning layer verifies this claim against authoritative sources, flagging discrepancies before the information is presented to decision-makers.
Logic-Based Constraint Satisfaction
Weave.AI’s symbolic reasoning engine applies deterministic logic to validate AI outputs, reducing the likelihood of hallucinations that arise from pattern-based errors. For instance, if an LLM recommends an investment that violates regulatory compliance guidelines, the symbolic layer applies logical constraints to filter out non-compliant recommendations, ensuring that all suggested actions adhere to established business rules.
Explainable AI (XAI) with Traceable Outputs
Weave.AI provides a transparent reasoning framework that enables users to trace how AI-generated insights are derived. This auditability ensures that enterprise decision-makers can confidently assess the validity of recommendations by reviewing the underlying data and logical inferences that informed the output. This feature is particularly critical in industries like finance and compliance, where verifiability is essential for regulatory adherence and risk management.
Real-World Impact: Safeguarding High-Stakes Decisions
By embedding a neuro-symbolic architecture into enterprise AI workflows, Weave.AI significantly reduces the risk of hallucinations while enhancing decision-making quality. For example:
Investment Strategy Validation: Weave.AI can analyze a company’s financial performance and market position while applying regulatory constraints and industry-specific benchmarks to validate investment recommendations.
Regulatory Compliance Audits: For compliance teams, Weave.AI ensures that internal policies are benchmarked against evolving regulations, identifying gaps or misalignments before they lead to regulatory penalties.
Delivering AI ROI While Mitigating Risk
For C-suite executives, the value proposition of Weave.AI extends beyond mitigating hallucinations—by ensuring that AI-generated insights are precise, verifiable, and aligned with enterprise objectives, Weave.AI enhances the ROI of AI initiatives while safeguarding the business from AI-related risks. As organizations scale their use of GenAI, adopting Weave.AI’s neuro-symbolic approach offers a pathway to unlocking transformative value without sacrificing trust, accuracy, or compliance.
Comments